Natt Fry, a renowned thought leader in the world of retail and analytics, published recently an article expounding the value and potential of learning models influencing business decision-making across industries over the next few years.
He quotes a Wall Street Journal article (paywall) published by Steven A. Cohen and Matthew W. Granade who claim that, “while software ate the world the past 7 years, learning models will ‘eat the world’ in the next 7 years.”
The article defines a learning model as a “decision framework in which the logic is derived by algorithm from data. Once created, a model can learn from its successes and failures with speed and sophistication that humans usually cannot match.”
Narrowing this down to the world of retail, Natt states, “if we believe that learning models are the future, then retailers will need to rapidly transform from human-learning models to automated-learning models.”
This, of course, comes with several challenges, one of which is the scarcity of easily consumable data for supervised learning algorithms to get trained on. This scarcity often results in a garbage-in-garbage-out situation and limits the ability of AI systems to improve in accuracy over time, or to generate meaningful output on a consistent basis.
Enabling Retailers Become More Model-Driven
As a provider of Competitive Intelligence as a Service to retailers and consumer brands, DataWeave uses highly trained AI models to harness and analyze massive volumes of Web data consistently.
Far too often, we’ve seen traditional retailers rely disproportionately on internal data (such as POS data, inventory data, traffic data, etc.) to inform their decision-making process. This isn’t a surprise, as internal data is readily accessible and likely to be well structured.
However, if retailers can harness external data at scale (from the Web — the largest and richest source of information, ever), and use it to generate model-driven insights, they can achieve a uniquely holistic perspective to business decision-making. Also, due simply to the sheer vastness of Web data, it serves as a never-ending source of training data for existing models.
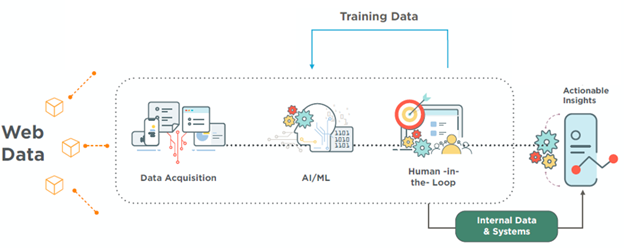
Web data is typically massive, noisy, unstructured, and constantly changing. Therefore, at DataWeave, we’ve designed a proprietary data aggregation platform that is capable of capturing millions of data points from complex Web and mobile app environments each day.
We then apply AI/ML techniques to process the data into a form that can be easily interpreted and acted on. The human-in-the-loop is an additional layer to this stack which ensures a minimum threshold of output accuracy. Simultaneously, this approach feeds information on human-driven decisions back to the algorithm, thereby rendering it more and more accurate with time.
Businesses derive the greatest value when external model-based competitive and market insights are blended with internal data and systems to generate optimized recommendations. For example, our retail customers combine competitor pricing insights provided by our platform with their internal sales and inventory data to develop algorithmic price optimization systems that maximize revenue and margin for millions of products.
This way, DataWeave enables retailers and consumer brands to utilize a unique model-based decision framework, something that will soon be fundamental (if not already) to business decision-making across industry verticals and global regions.
As AI-based technologies become more pervasive in retail, it’s only a matter of time before they’re considered merely table stakes. As summarized by Natt, “going forward, retailers will be valued on the completeness of the data they create and have access to.”
If you would like to learn more about how we use AI to empower retailers and consumer brands to compete profitably, check out our website!
Read Natt’s article in full below:
Steven A. Cohen and Matthew W. Granade published a very interesting article in the Wall Street Journal on August 19, 2018 — https://www.wsj.com/articles/models-will-run-the-world-1534716720
Their premise is that while software ate the world (Mark Andreessen essay in 2011, “Why Software is Eating the World”) the past 7 years, learning models will “eat the world” in the next 7 years.
A learning model is a decision framework in which the logic is derived by algorithm from data. Once created, a model can learn from its successes and failures with speed and sophistication that humans usually cannot match.
The authors believe a new, more powerful, business opportunity has evolved from software. It is where companies structure their business processes to put continuously learning models at their center.
Amazon, Alibaba, and Tencent are great examples of companies that widely use learning models to outperform their competitors.
The implications of a model-driven world are significant for retailers.
Incumbents can have an advantage in a model-driven world as they already have troves of data.
Going forward retailers will be valued on the completeness of the data they create and have access to.
Retailers currently rely on the experience and expertise of their people to make good decisions (what to buy, how much to buy, where to put it, etc.).
If we believe that learning models are the future then retailers will need to rapidly transform from human-learning models to automated-learning models, creating two significant challenges.
First, retailers have difficulty in finding and retaining top learning-model talent (data scientists).
Second, migrating from human-based learning models to machine-based learning models will create significant cultural and change management issues.
Overcoming these issues is possible, just as many retailers have overcome the issues presented by the digital age. The difference is, that while the digital age has developed over a 20 year period, the learning-model age will develop over the next 7 years. The effort and pace of change will need to be much greater.